AI Chip Center for Emerging Smart Systems (ACCESS) is proud to announce that its latest research paper, titled "A 28nm 0.22μJ/Token Memory-Compute-Intensity-Aware CNN-Transformer Accelerator with Hybrid-Attention-Based Layer-Fusion and Cascaded Pruning for Semantic-Segmentation," has been accepted and presented at the International Solid-State Circuits Conference (ISSCC) 2025, the premier global forum often referred to as the "Olympics of Chip Technology." Co-authored by ACCESS professors, graduate students, and the R&D team, this milestone marks the first AI computing chip paper from Hong Kong to be published at this prestigious conference, highlighting international recognition of ACCESS's innovative capabilities in fundamental research and design implementation.
Recent advancements in hybrid models combining Convolutional Neural Networks (CNN) and Transformers have demonstrated remarkable progress in semantic segmentation tasks, with applications in autonomous driving and embodied intelligence. However, hardware acceleration for such models faces significant challenges, including increased external memory access (EMA) due to large token lengths and impractical traditional layer-fusion, as well as high computational costs stemming from extremely low sparsity in the segmentation head. To address these challenges, the ACCESS team developed a high-efficiency CNN-Transformer accelerator architecture, featuring three key innovations:
1. Hybrid Attention Processing Unit: Integrates memory-efficient linear attention with accurate vanilla attention, reducing EMA by 22.05x.
2. Layer-Fusion Scheduler: Optimizes layer-fusion between various attentions and convolution through out-of-order execution, boosting energy efficiency by 1.45x.
3. Cascaded Fmap Pruner: Unifies attention-convolution Fmap pruning by weight decomposition, improving 76.1% Fmap sparsity and reducing computation by 6x.
Building upon these innovations, ACCESS designed and taped-out the 28nm commercial-grade test chip, AC-Transformer. This chip fully realizes programmable end-to-end inferences for CNN and Transformer models, achieving extraordinary energy efficiency. On the SegFormerB0 model, the AC-Transformer chip consumes merely 0.22μJ per token, resulting in a system-level energy efficiency improvement of 3.86-10.91 times compared with state-of-the-art results.
The success of this research has garnered high acclaim from the international academic community, showcasing the dedication of the professors, graduate students, researchers, and engineers involved in the project. It also highlights the power of collaborative innovation in advancing AI computing architecture. Moving forward, ACCESS will continue to advance intelligent computing technologies, explore more efficient and advanced AI chip architectures, and contribute further groundbreaking innovations to the industry.
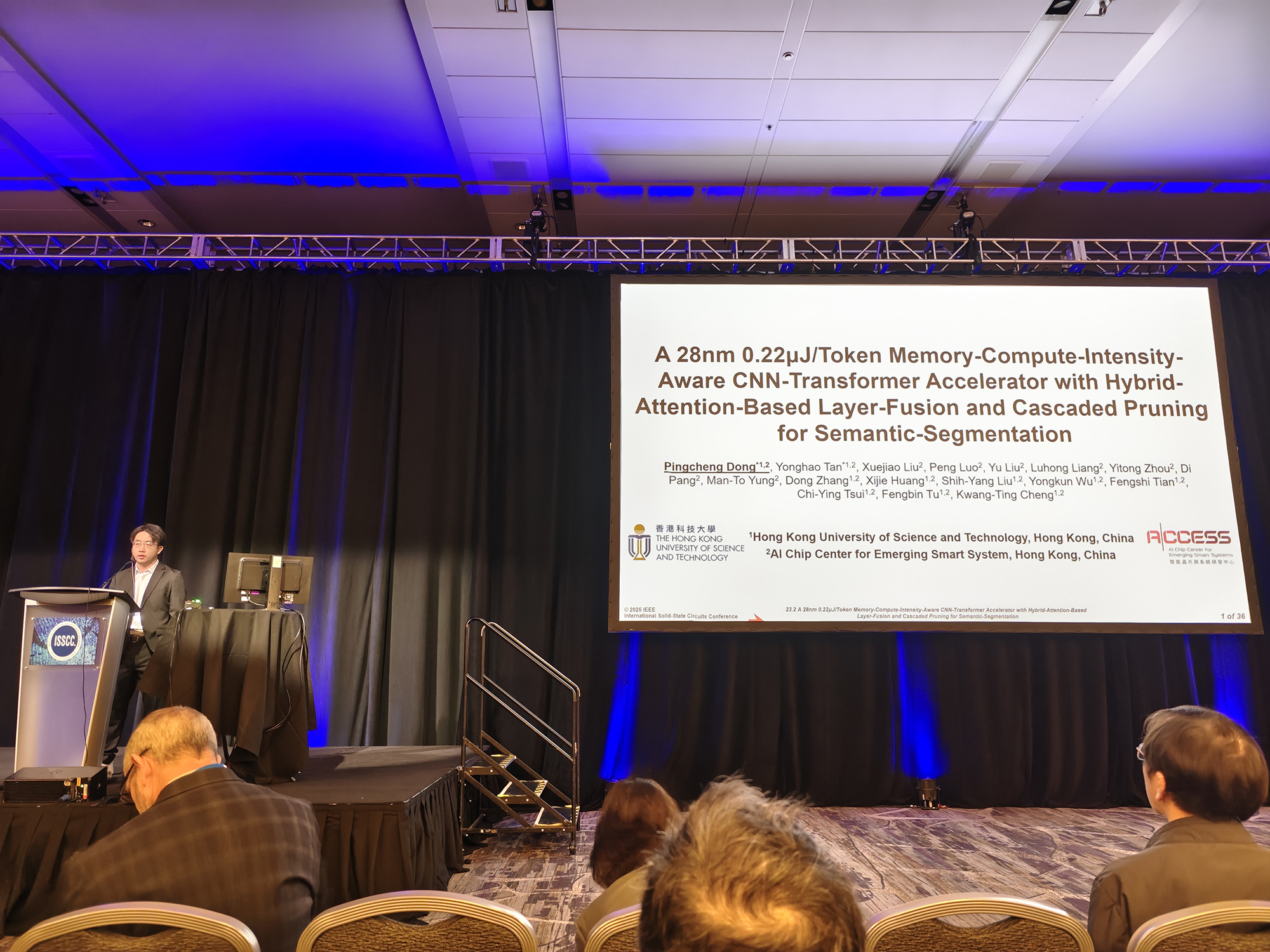
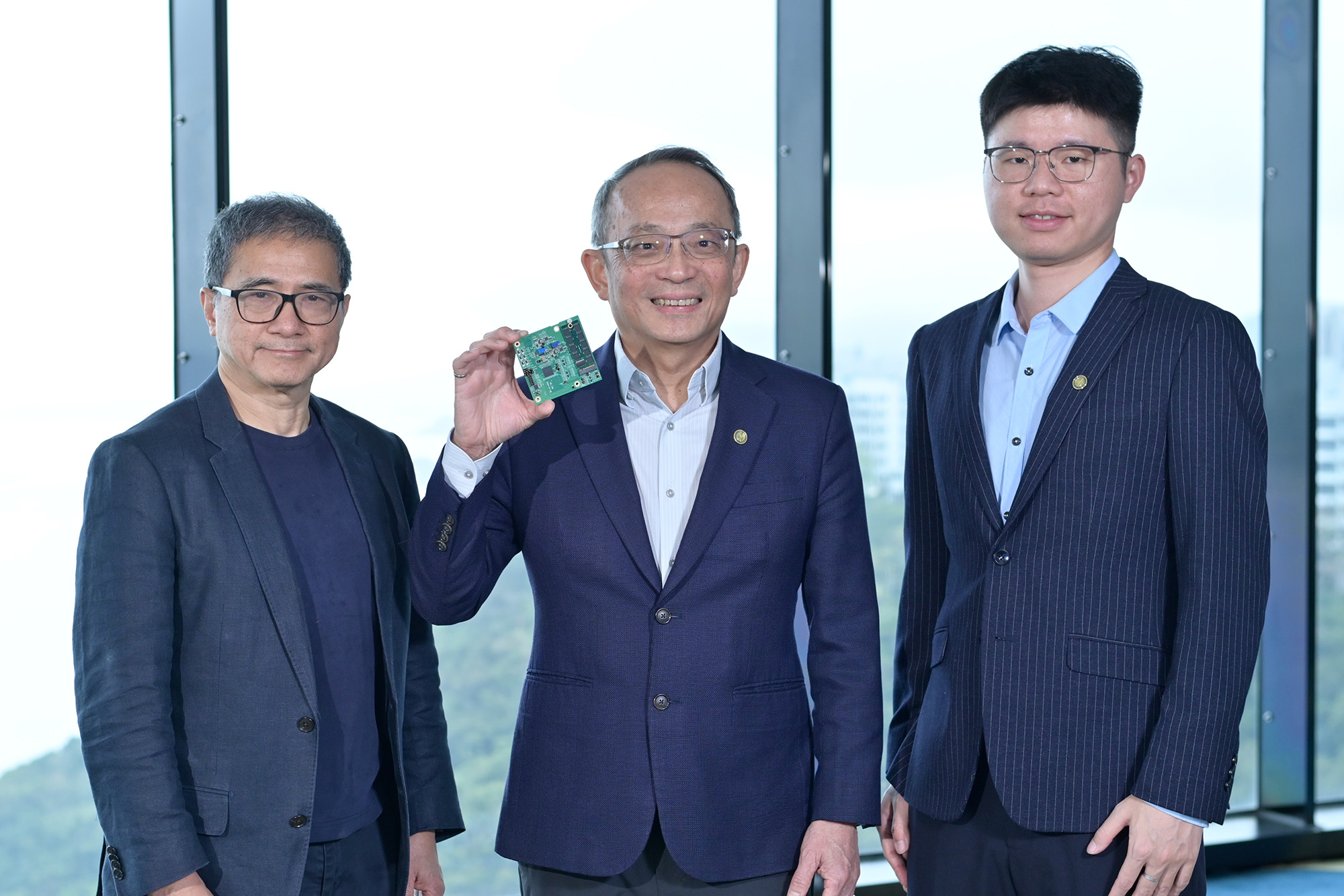
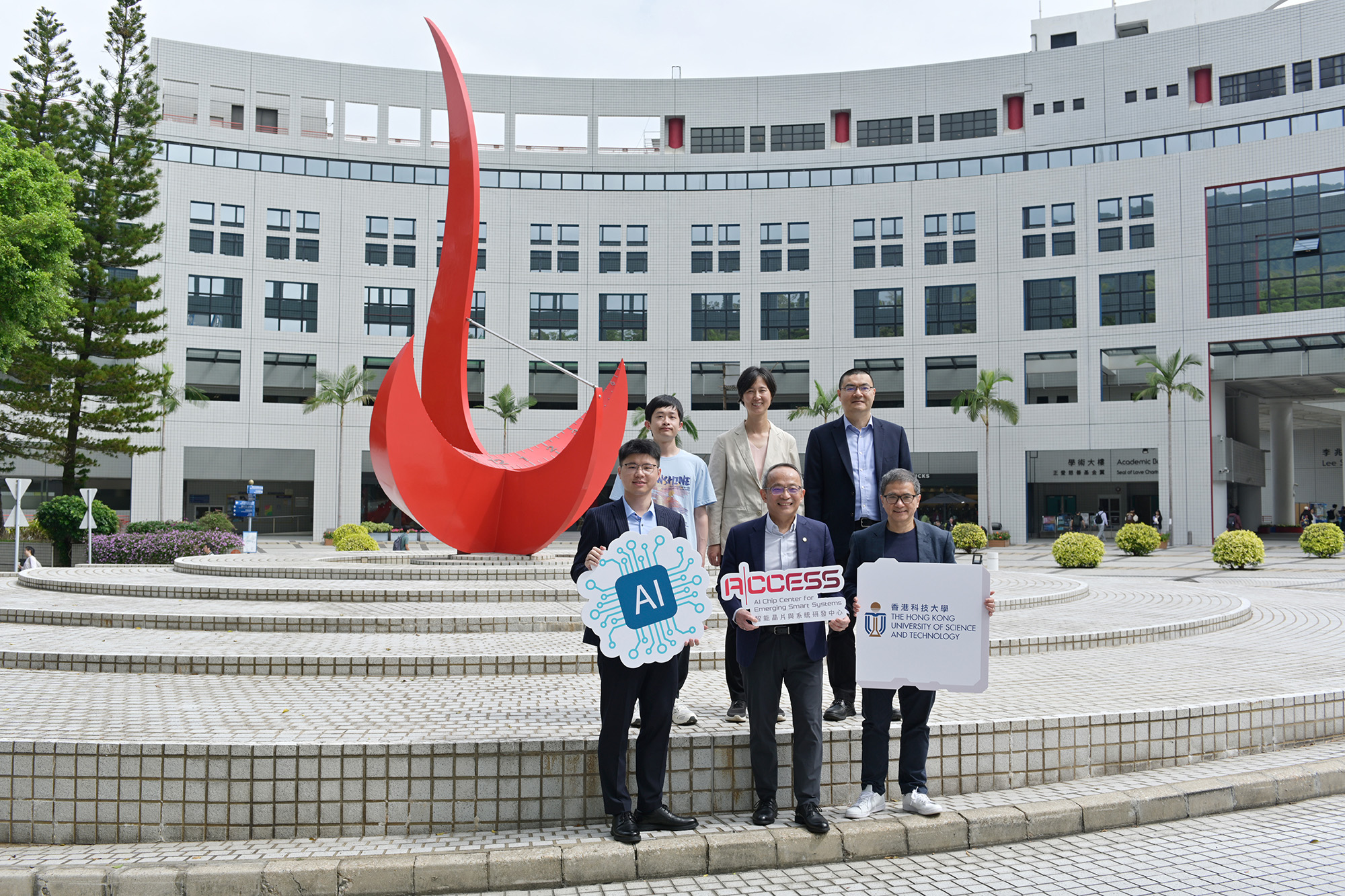
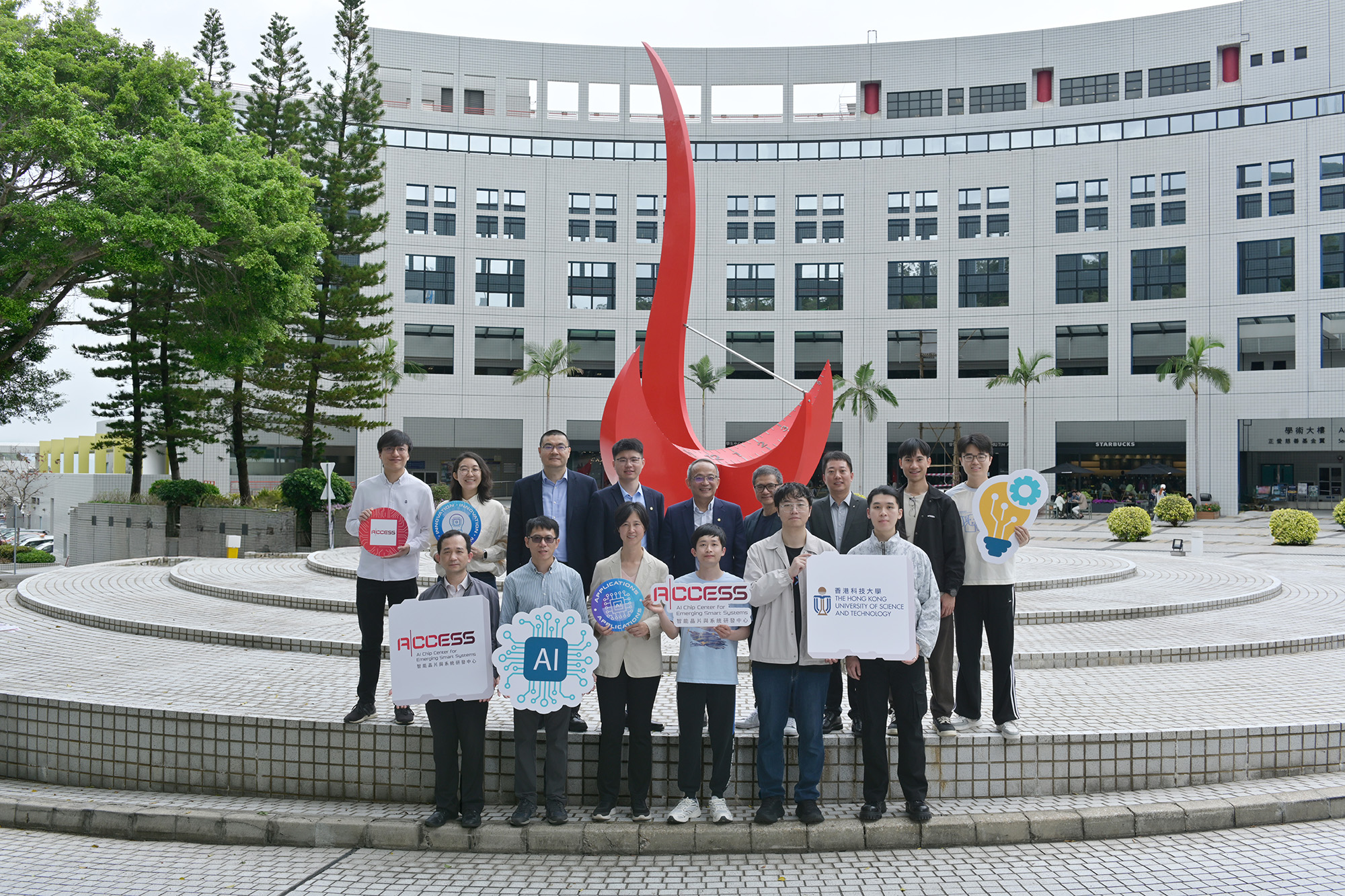
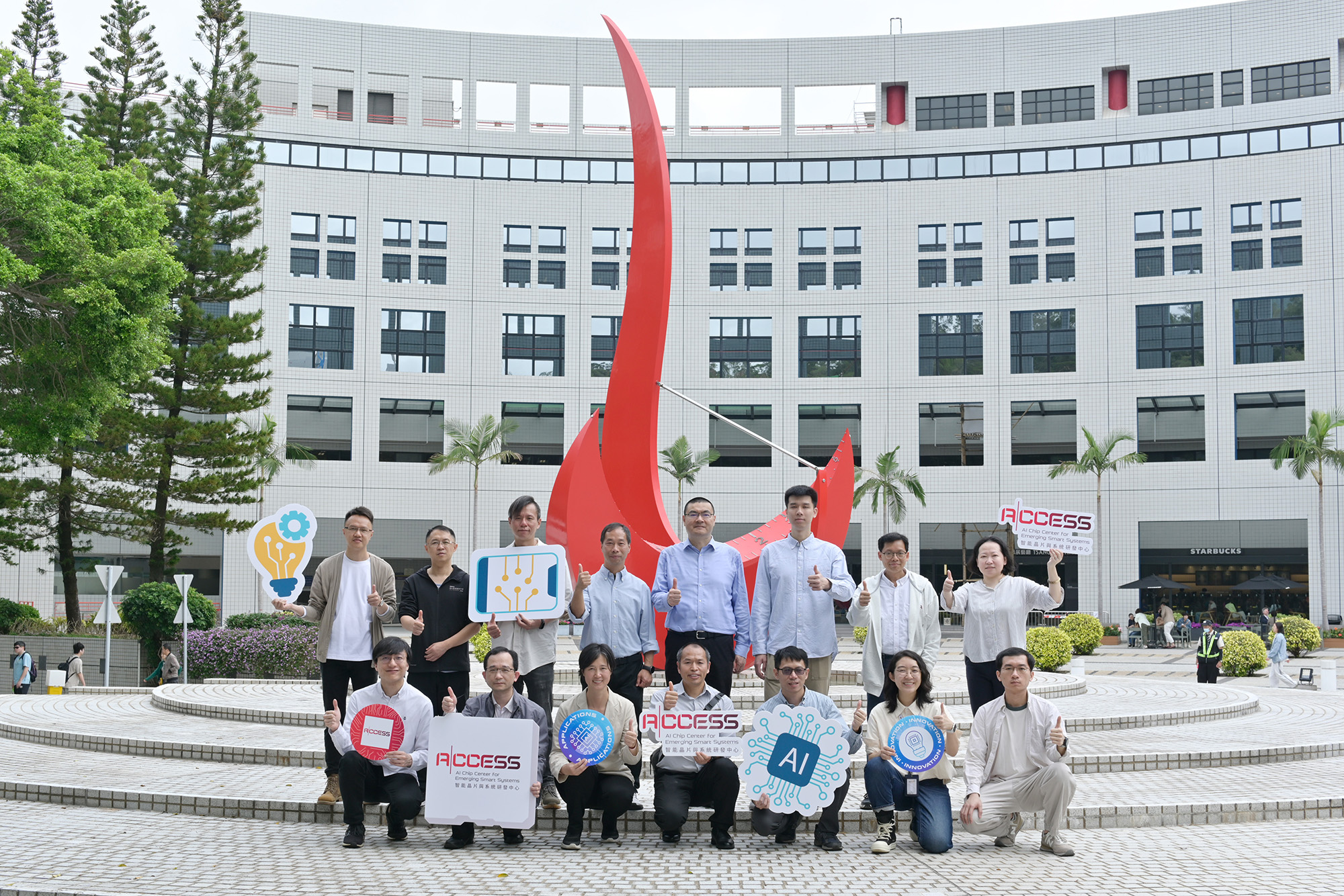
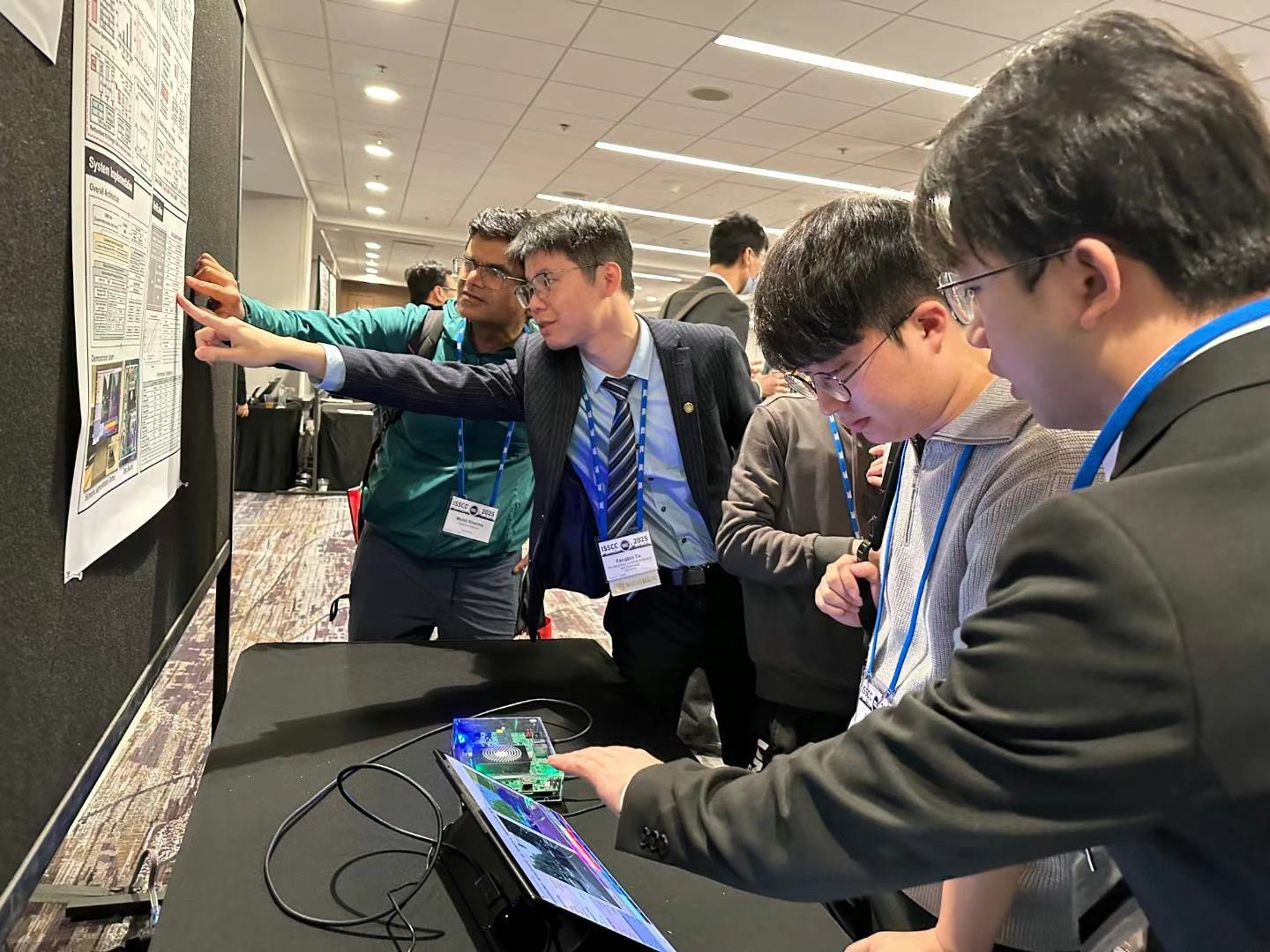